A brief primer on scientific and mathematical notations
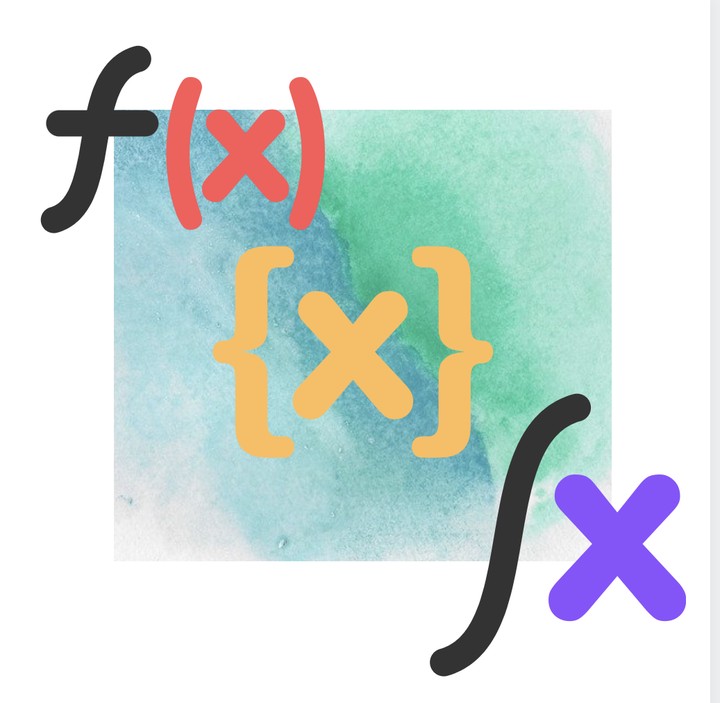
As I finished writing the final draft of my first first author paper, survClust, there were a lot of other firsts! In my opinion writing the methods and a crisp conclusion and discussion were the difficult parts.
Below, I share my notes that really came in handy while I was writing the methods section of my manuscript.
What this is?
Notes on how to describe a statistical methodology. Some basic rules and notations that you should keep in mind.
Scientific notations
Random variables are usually written in uppercase roman letters: \(X,Y\), etc.
Probability density functions (pdfs) and probability mass functions are denoted by lowercase letters, e.g. \(f_{(x)}\), or \(f_{X}(x)\).
Cumulative distribution functions (cdfs) are denoted by uppercase letters, e.g. \(F(x)\), or \(F_{X}(x)\).
Let's summarize the above three points with an example -
A random variable \(X\) has density \(f_{X}\) as follows -
\[ Pr[a\leq X\leq b]=\int _{a}^{b}f_{X}(x)\,dx\]
Hence, if \(F_{X}\) is the cumulative distribution function of \(X\) then:
\[F_{X}(x)=\int _{-\infty }^{x}f_{X}(u)\,du,\]
and
\[f_{X}(x)={\frac {d}{dx}}F_{X}(x).\]
Now, let's go over some quick statistical nitty-gritties:
Greek letters \(\theta, \beta\) are commonly used to denote unknown parameters.
Placing a hat, or caret, over a true parameter denotes an estimator of it, e.g., \(\widehat {\theta }\) is an estimator for \(\theta\) .
Building on the above point the sample mean, variance and correlation coefficient are denoted as \(\bar{x}, s^2, r\) respectively. On the other hand population parameters are represented as follows - population mean \(\mu\), population variance \(\sigma^2\), and population correlation as \(\rho\)
Finally most of the time you will need to know the following writing notions while drafting the methods section of your manuscript -
Input or independent variables are denoted by \(X\), output or dependent variables are denoted by \(Y\), and qualitative outputs by \(G\).
If \(X\) is a vector, annotate its values by subscripts \(X_j\)
Observed values are written in lowercase; hence the \(i^{th}\) observed value of \(X\) is written as \(x_i\), where \(x_i\) is a scalar or vector.
Matrices are represented by bold uppercase letters; for example a matrix \(\textbf{X}\), with dimensions \(N\) x \(p\) i.e a set of \(N\) input \(p\)-vectors. In general, vectors will not be bold, except when they have \(N\) components; Note that all vectors are assumed to be column vectors.
Let's break it down with an example -
Given a vector of inputs \(\textbf{X}^T = (X_1,X_2,...,X_p)\), we predict the output \(\textbf{Y}\) via a simple linear regression -
\[\hat{\textbf{Y}} = \hat\beta_0 + \sum_{n=1}^{p} \textbf{X}_{j}\hat\beta_{j}\] Or writing this in a vector form as an inner product - \(\hat{\textbf{Y}} = \textbf{X}^T\hat\beta\) To solve this we need to estimate a value of \(\beta\) such that it minimizes the Residual Sum of Squares or RSS as follows -
\[RSS(\beta) = \sum_{i=1}^{N} (y_i - x_{i}^T\beta)^2\]
Or in matrix notation we can write it as,
\[RSS(\beta) = (\textbf{y} - \textbf{X}\beta)^T(\textbf{y} - \textbf{X}\beta)\] where \(\textbf{X}\) is an \(N × p\) matrix with each row an input vector, and \(\textbf{y}\) is an \(N\)-vector of the outputs. See how \(\textbf{y}\) is in bold in the above question.
Or take one of your favorite papers, and try to go over its methods section to iron and figure out other key details!